You have arrived… are we there yet?
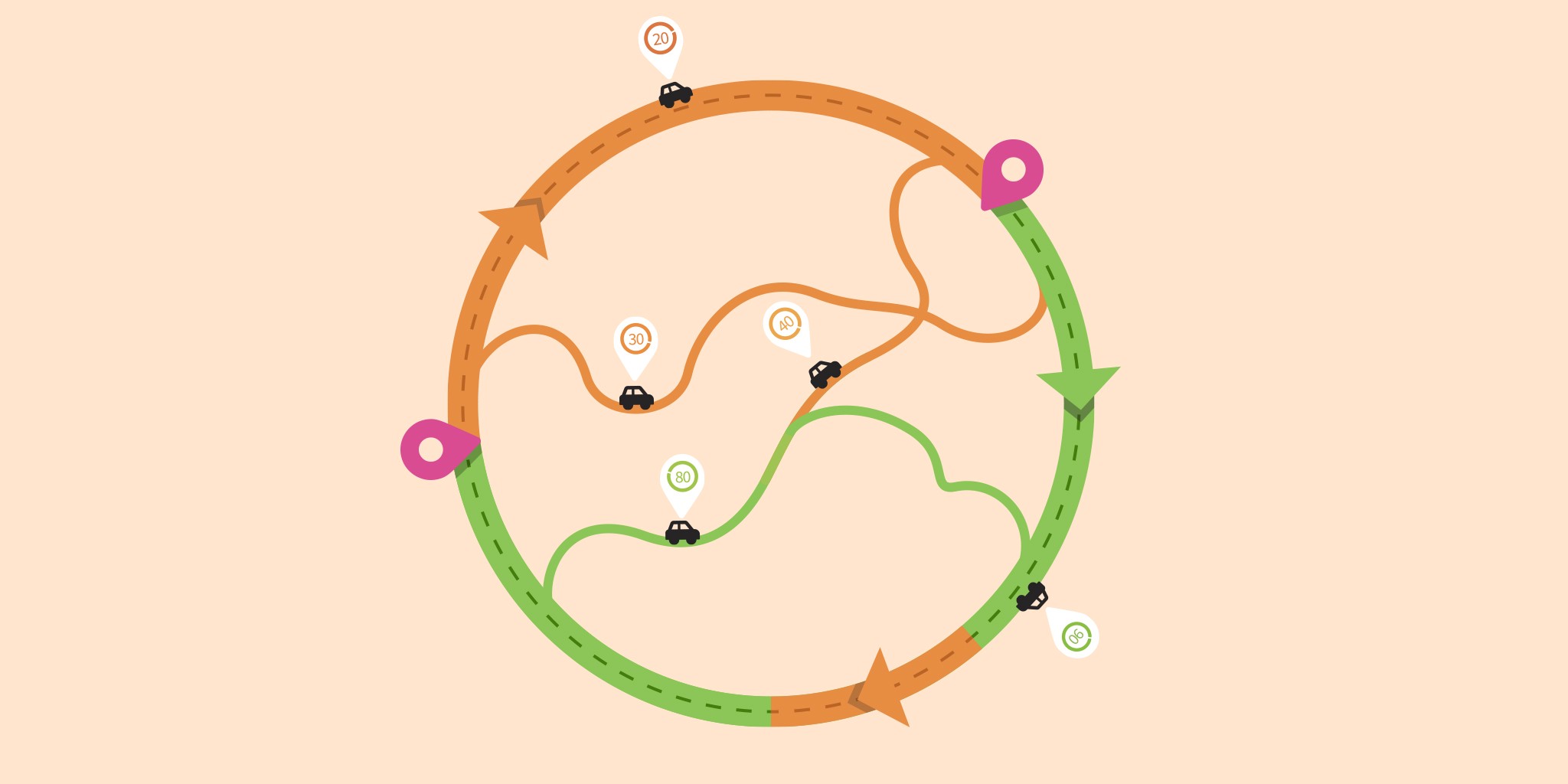
Do you remember life before turn by turn navigation? It really wasn’t that long ago we happily engaged in the ancient practices of scribbling directions on a scrap piece of paper, printing them out before leaving the house, or even pulling over and asking a fellow human how to get there from here. Today, though, we only ask our machines for directions, and when we reach our destination, they offer us some form of this outlandishly encouraging message :
“You have arrived!” Yes, computer, compared to the old days, we have arrived!On second thought, have we really arrived? You said it would take 30 minutes to get there and now you’re saying 40? What’s the deal? Yes, I know I’m starting to sound like my kids, asking “Are we there, yet?”
Here’s the conundrum — the data underneath the engine predicting the best route is constantly changing. With ongoing construction projects and new roads opening, not to mention this morning’s traffic, the data under these apps is dynamic. Without constantly updated data, these apps become no better than static printed out directions.
Augmented writing shares a similar challenge. The language you use has the power to change how people respond. Like road systems, though, language is constantly changing over time. As language changes, your written communication now includes updated and outdated language which in turn yields changing outcomes. With these cyclical complexities, static data like word lists and rule checkers quickly become irrelevant. So how do platforms built on dynamic data stay current? The answer is learning loops.
A learning loop is any cycle where data is continually fed into a predictive engine that helps that engine make more accurate predictions based on the current data. For example, Textio’s data model for job posts is constantly ingesting the latest job post language and associated outcomes which improves the predictions and suggestions for what you are writing today.
Without this learning loop, you might as well take a list of words that some good intentioned human decided might prevent bias or improve engagement and hope that list stays true forever — much like our outdated printed map. When Textio ingests new data and new language patterns emerge, Textio gets smarter and your Textio Scores and tone in your library will change. Here are a few real life examples of changing language patterns that Textio has uncovered in recent months.
Data has long confirmed strong job posts balance “we” statements that describe your company and “you” statements that directly address job seekers, recent data identified a shift in the balance.
Today’s data shows that instead of a strict one-to-one ratio of “you” phrases and “we” phrases in a job post, the best performing job posts have a slightly higher ratio of “we” statements.Utilizing statements such as “our team” and “we value” over “you” statements like “You love learning,” improves the performance of your job post in today’s market.
Here is another recent data point that underscores the importance of writing job posts to engage real humans. The word “candidate” has historically correlated with a slower time to fill.
Current data from today’s hiring market shows using “candidate” once or twice has minimal impact on time to fill, but used more than 3 times correlates with a longer time to fill.
Textio’s learning loop regularly uncovers new phrases that uniquely impact a specific company as well as impacting global English job posts. For example, the phrase “attention to detail” has recently been identified as a phrase that fills job posts faster. There could be many cultural reasons to explain why this is happening, but Textio’s data loop is capturing the fact that it is happening. The data doesn’t lie.
These examples are from language in global English job posts, but there are localized learning loops, too. In fact, every Textio customer has their own unique learning loop in their library feeding Textio Flow. Every edit you make to any document in your central library has an impact on the next colleague that writes a job post. If making one edit affects the learning loop, consider the benefit you are bringing your team every time you create new original job posts with a high score and neutral tone and mark it as shared or finished. You are building new roads for efficiency and creativity for your colleagues to generate candidate communication content with a single keystroke. Well done!
Learning loops are required for dynamic data. Are they helpful for us humans? Have learning loops for turn by turn navigation diminished the sense of adventure on a road trip? I’d say the data has even freed us to be more adventurous! One day, augmented writing will be so common, that typing in a text box without it will feel similar to scribbling directions on a napkin, but don’t worry — learning loops in augmented writing will not diminish the creativity of writers. They will invite us to new levels of creativity to define what great communication looks like. After all, we humans are dynamic, too.
Happy driving and happy writing!